32
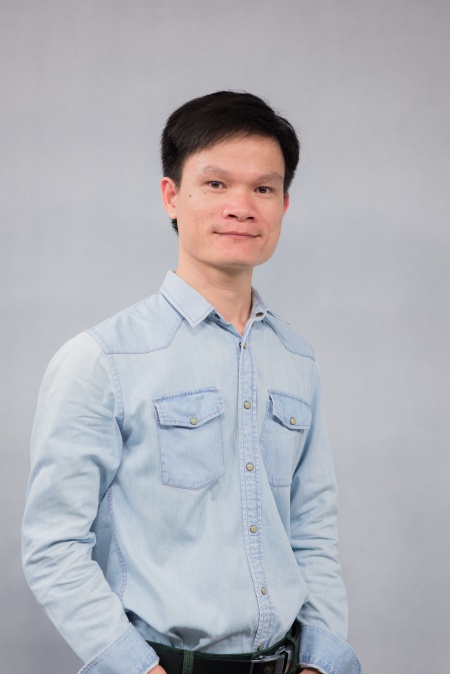
Than Quang Khoat
Head, Data Science Laboratory
Associate Professor, Department Of Computer Science
Ph.D. (Knowledge Science, Japan Advanced Institute of Science and Technology, 2013)
M.S. (Computer Science, Hanoi University of Science and Technology, 2009)
B.S. (Applied Mathematics & Informatics, Vietnam National University, 2004)
Email: khoattq@soict.hust.edu.vn
Web: https://users.soict.hust.edu.vn/khoattq/
Research Areas
- Artificial Intelligence
- Data Science
- Computer Science
Research Interests
- Machine Learning
- Representation Learning
- Continual Learning
- Recommender System
- Online Advertisement
- Big Data
Profile
Khoat Than is currently Director of Data Science Laboratory, and Assistant Professor at Department of Information Systems, SOICT, HUST. He received Ph.D. (2013) from Japan Advanced Institute of Science and Technology. He often joins the Program Committees of various leading conferences in the areas of artificial intelligence and data science, including ICML, NIPS, IJCAI, ICLR, PAKDD, ACML. His research has been being supported from various funding sources including ONRG (US), AFRL (US), ARL (US), NAFOSTED (VN), MOET (VN).
Publications
- Cuong Ha-Nhat, Van-Dang Tran, Linh Ngo Van, Khoat Than, “Eliminating overfitting of probabilistic topic models on short and noisy text: The role of Dropout”, International Journal of Approximate Reasoning, Elsevier, 2019.
- Hoa Le Minh, Son Ta Cong, Quyen Pham The, Linh Ngo Van, Khoat Than, “Collaborative Topic Model for Poisson distributed ratings”, International Journal of Approximate Reasoning, Volume 95, Pages 62-76, Elsevier, 2018.
- Tu Vu, Xuan Bui, Khoat Than, Ryutaro Ichise, “A flexible stochastic method for solving the MAP problem in topic models”, Computacón y Sistemas journal, 2018.
- Ngo Van Linh, Nguyen Kim Anh, Khoat Than, Chien Nguyen Dang, “An Effective and Interpretable Method for Document Classification”, Knowledge and Information Systems, Springer, 2017.
- Khoat Than, Tu Bao Ho, and Duy Khuong Nguyen, “An effective framework for supervised dimension reduction”. Neurocomputing, vol .139, pages 397-407, Elsevier, 2014.
- Khoat Than and Tu Bao Ho, “Modeling the diversity and log-normality in data”. Intelligent Data Analysis: An International Journal, vol. 18, no.6, 2014.
- Linh The Nguyen, Linh Van Ngo, Khoat Than, and Thien Huu Nguyen, “Employing the Correspondence of Relations and Connectives to Identify Implicit Discourse Relations via Label Embeddings”, In Proceeding of the Association for Computational Linguistics (ACL), 2019.
- Khoat Than, Xuan Bui, Tung Nguyen-Trong, Khang Truong, Son Nguyen, Bach Tran, Linh Ngo Van, and Anh Nguyen-Duc “How to make a machine learn continuously: a tutorial of the Bayesian approach”, Proc. SPIE 11006, Artificial Intelligence and Machine Learning for Multi-Domain Operations Applications, 110060I (May 2019); doi: 10.1117/12.2518860
- Duc-Anh Nguyen, Kim Anh Nguyen, Linh Ngo, Khoat Than, “Keeping priors in streaming Bayesian learning”, In Proceedings of the 21st Pacific-Asia Conference on Knowledge Discovery and Data Mining (PAKDD). Lecture Notes in Computer Science, Springer, 2017.
- Tung Doan and Khoat Than, “Sparse Stochastic Inference with Regularization”, In Proceedings of the 21st Pacific-Asia Conference on Knowledge Discovery and Data Mining (PAKDD). Lecture Notes in Computer Science, Springer, 2017.
Awards & Honours
- Student Best Paper: IEEE International Conference on Research, Innovation & Vision for the Future, 2008
- Best Student Paper: The 10th IEEE RIVF International Conference on Computing and Communication Technologies, Hanoi, 2013.
- Monbukagakusho Scholarship: Japanese Government MEXT Scholarship, 10/2009-9/2013
- Travel grants: NEC C & C Foundation (2010), Japan Association for Mathematical Sciences (2012), NAFOSTED (2016); ARL (2019)
Teaching
- Artificial Intelligence
- Machine Learning
- Introduction to Data Science
- Machine Learning for Big Data
Current Projects
- Learning an effective representation for the hidden semantics (Role: Dirrector / Principle Investigator)
- Multi-tasking Evolutionary Algorithms for Optimizing Artificial Neural Network and Graph-based Models (Role: Investigator)
- Develop new transition-metal-rare-earth materials for permanent magnets by machine learning Models (Role: Investigator)